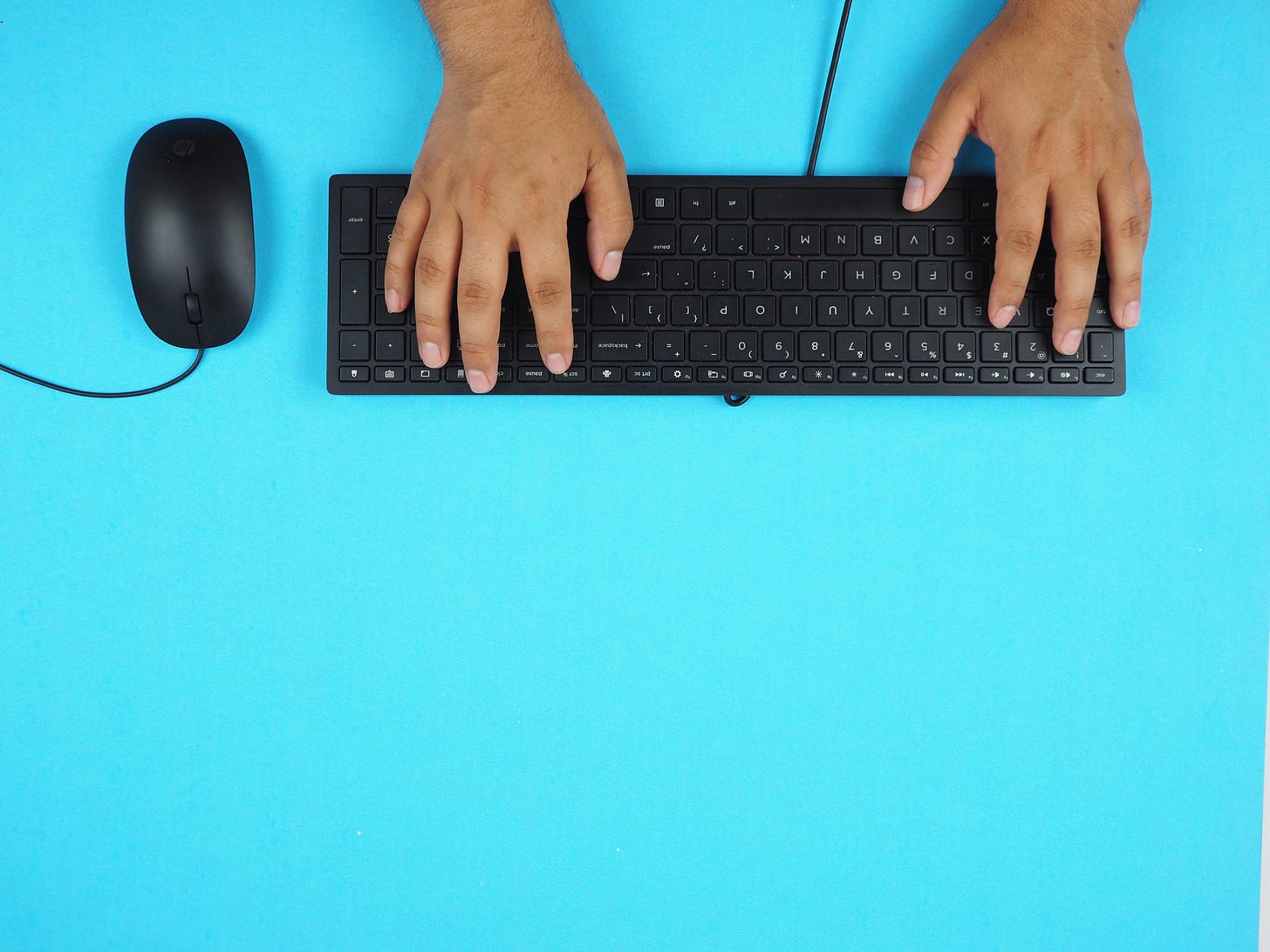
Measuring user experience (UX) is important to understand how successful applications and systems are in reaching their goals. Two self-report methods — questionnaires and interviews — comprise more than half of the data collection methods used for UX research. More specifically, a review by Bargas-Avila & Hornbæk found that 53% of all UX research is accomplished with questionnaires, 20% of all studies use semi-structured interviews, and 15% make use of focus groups.
Self-report techniques can be helpful when conducting UX research, but they do suffer from a number of limitations...
Self-report techniques allow us to gain some insight into the relationship between user characteristics, their needs and preferences, their behaviour, and their experience, but they do suffer from a number of limitations. For example, social-desirability bias and self-presentation motivations can make users purposefully change their answers and lie to researchers. This is more likely to occur when their answers are believed to violate social norms or affect one’s self-image negatively (e.g., users might not be willing to admit to not understanding a feature). Another limitation of self-report measures is the fact that people are not always fully aware of all their feelings and what triggers them. Unconscious motives (i.e., emotions, implicit attitudes) — which people are not always aware of — need to also be taken into account in order to fully understand user experience. This challenge is not limited to UX research; psychologists have been looking for ways to overcome these limitations for years.
Unconscious motives (i.e., emotions, implicit attitudes) need to be taken into account in order to fully understand user experience.
Implicit measures are one of the techniques that have been developed to solve this issue. Implicit measures are cognition measurement procedures whose intent is to capture automatic psychological attributes that respondents are unwilling or unable to report. More specifically, implicit methods allow us to measure cognitions or experiences without the need for introspection; there is no need for the individual to recall their experience, understand, or verbalise it. In fact, these measures allow us to examine attitudes or evaluations the individual might not even be aware of. As a result, our findings are less likely to be influenced by factors like social desirability and self-presentation.
The Implicit Associate Test (IAT), developed by Greenwald, McGhee, and Schwartz is probably the most well-known implicit measuring method (for a critical review see this). The IAT indirectly measures the strength of associations between concepts (e.g., black people, gay people) and evaluations (e.g., good, bad) or stereotypes (e.g., athletic, clumsy). The main idea is that making a response is easier when closely related items share the same response key. For example, having an implicit preference towards Category A would lead a participant to respond faster when concepts related to being Category A share the same response as positive evaluations. If you’re curious and want to find out more about the IAT check out the Project Implicit website.
The IAT can provide some insight into unconscious processes that are proved to influence our behaviour, such as emotions, feelings, and stereotypes (e.g., what we fight physically attractive). Only a small number of studies have investigated the possibility of using implicit measures in measuring UX by using modified versions of the IAT and comparing the findings to explicit measures and previous research.
The first study using the IAT in the context of UX research was conducted by Strasser, Weiss, and Tscheligi and aimed to measure participants’ attitudes towards robots and their user experience. The implicit measure revealed a negative tendency towards a certain type of robots, while the explicit measures did not show this tendency. This finding suggests that implicit measures can give us additional insight into UX.
More recently, Actis-Grosso and colleagues recruited 36 participants and asked them to evaluate a conversational chatbot prototype, whose role was to pre-select candidates for a job and to arrange a date for the job interview. The researchers manipulated between-participants the chatbot’s gender (male vs. female) and the tone of voice (formal vs. informal).
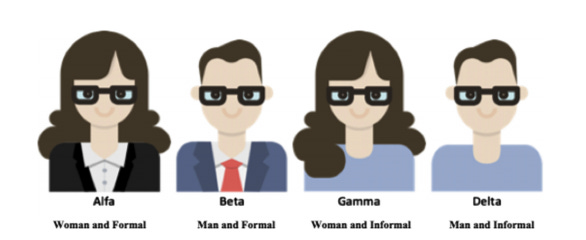
After interacting with the chatbot, participants filled in a questionnaire explicitly measuring the user experience’s evaluation and implicit attitudes towards the chatbot (modified IAT). The results showed an implicit preference towards the informal version of the chatbot, as revealed by the IAT scores, whereas no differences emerged on the explicit measures.
What does this mean for practitioners?
Implicit measures are being used by marketing professionals and there is some evidence suggesting they can be useful under certain circumstances. In For example, research has shown that people are likely to choose the implicitly preferred brand over the explicitly preferred one when choices were made under time pressure.
If we want to better understand UX, implicit attitudes should be measured. This would allow us to tap into the role of emotions, beliefs, and feelings that the user is not aware of or able to verbalise (due to bias). It is important to consider that our emotions are triggered not only by our conscious evaluations of a given situation but also by implicit attitudes such as social rules, prejudices, stereotypes, and expectations. Using measures like the IAT in combination with explicit measures (e.g., interviews, surveys), it would be possible to investigate the role of unconscious beliefs and stereotypes in shaping UX.
The use of the IAT as a measure for UX, however, needs further research. Preliminary results are promising and show that that IAT could be an effective tool for measuring implicit attitudes towards a chatbot or a robot. If you are a UX researcher in these fields, it might be worth considering this. More research is needed to establish the usefulness of the IAT in non-human-like products and interfaces.
References
Actis-Grosso, R., Capellini, R., Ghedin, F., & Tassistro, F. (2021, July). Implicit Measures as a Useful Tool for Evaluating User Experience. In International Conference on Human-Computer Interaction (pp. 3-20). Springer, Cham.
Allen, M. (2017). The sage encyclopedia of communication research methods (Vols. 1-4). Thousand Oaks, CA: SAGE Publications, Inc doi: 10.4135/9781483381411
Bargas-Avila, J. A., & Hornbæk, K. (2011, May). Old wine in new bottles or novel challenges: a critical analysis of empirical studies of user experience. In Proceedings of the SIGCHI conference on human factors in computing systems (pp. 2689-2698).
Friese, M., Wänke, M., & Plessner, H. (2006). Implicit consumer preferences and their influence on product choice. Psychology & Marketing, 23(9), 727-740.
Rezaei, A. R. (2011). Validity and reliability of the IAT: Measuring gender and ethnic stereotypes. Computers in human behavior, 27(5), 1937-1941.Chicago
Strasser, E., Weiss, A., & Tscheligi, M. (2012, March). Affect misattribution procedure: an implicit technique to measure user experience in hri. In Proceedings of the seventh annual ACM/IEEE international conference on Human-Robot Interaction (pp. 243-244).